Fully automated image processing has been improving over the years and has saved experts time and effort in their daily work, replacing time-consuming and expensive manual methods. But does the efficiency of these automated methods always have to compete with the accuracy of expert’s manual intervention? Here we want to present a hybrid automated and manual pipeline to produce the best results in an easy and traceable way.
Refining fully automated processing
A fully automated processing pipeline in the cloud allows researchers and clinicians to dedicate their attention to the crucial facet in medical imaging: the results. It provides the desired output with a click of a button, allowing a quicker analysis of the input data. But depending on the objectives and expectations, automated processing tools outputs are not always accurate enough. This can make experts less confident in conclusions drawn from them, lead to frustration, and ultimately hinder progress in the research.
Some automated pipelines consist of different processing steps, each step taking the outputs of the previous step as input, towards achieving a specific outcome, for example a full brain regions segmentation. Such tool structure could allow a user to inspect the quality on each step. A protocol describing what should be corrected and under which circumstances should be in place, taking into account the effort and the level of accuracy required to reach the final goal. Then, a decision to tune or not the intermediate results on-premise is taken and the workflow is restarted using the edited files as input.
In the process described in the previous paragraph, the user has refined the automated processing increasing the accuracy at particular steps, which leads towards an improvement of the final results.
QMENTA Platform hybrid process
This iterative hybrid process can be integrated in any of the QMENTA tools in a very simple way.
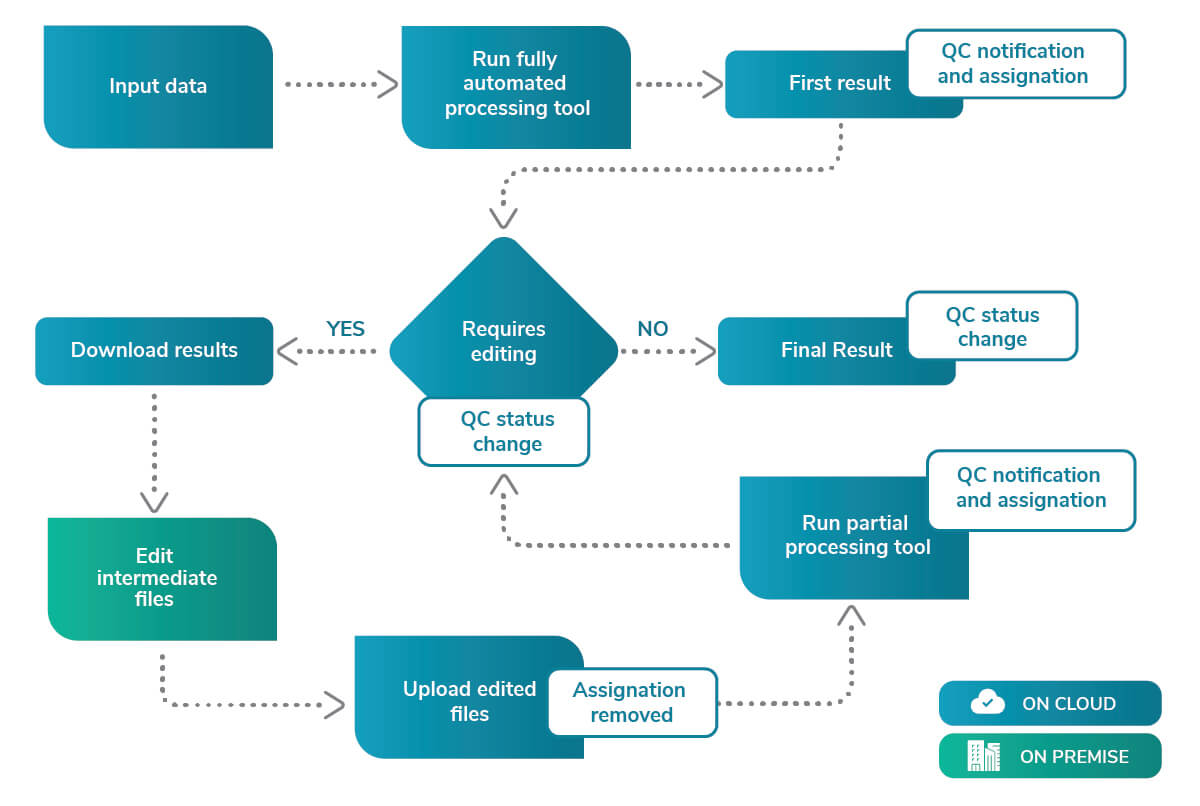
Overview of the iterative process.
On the first run, the tool will process the input data in a fully automated way. The results are accessible with the click of a button in the Analyses list, and the user can download the results to perform an on-premise quality control.
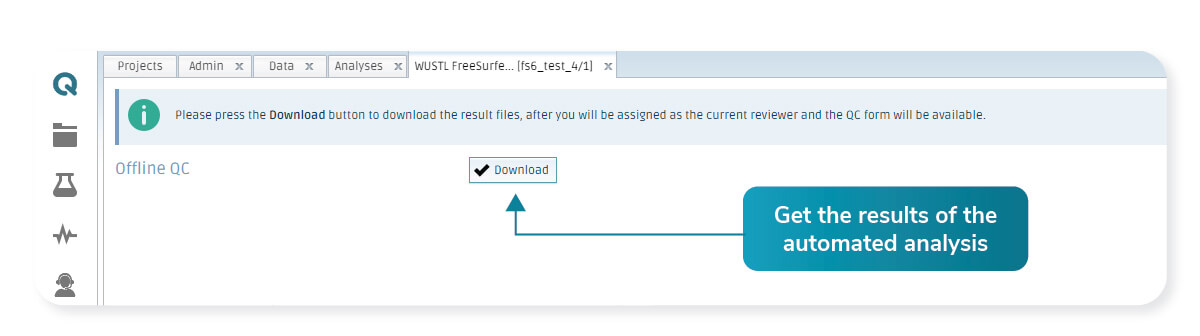
Platform interface allows you to download all the files generated by the fully automated tool.
The results are assessed on-premise and a decision on the quality is made. On the platform’s next step, the user can select the QC status of the analyzed results. It can be one of the following options:
1. PASS: The results produced are meeting the expectations.
2. FAIL needs reprocessing: The results can be tuned, and a new run is going to be performed using the edited files.
3. QUARANTINED: The processed data produced results that cannot be further improved by editing, and the session is going to be discarded.
4. FAIL: The processed data produced results that cannot be further improved by editing, and the session is going to be discarded.
An additional text box allows the user to add any other comment that is required to complete the information about the quality assessment carried out.
If option 2 is selected, a new button to upload the edited results is enabled. After the files are edited by the expert, they can be uploaded and will replace the already existing results. The tool will ask the user if they want to restart using the uploaded files. The platform will restart the process using the new uploaded set. However, it will run from an intermediate step where the changes will be taken into consideration, taking less time than the first full run.
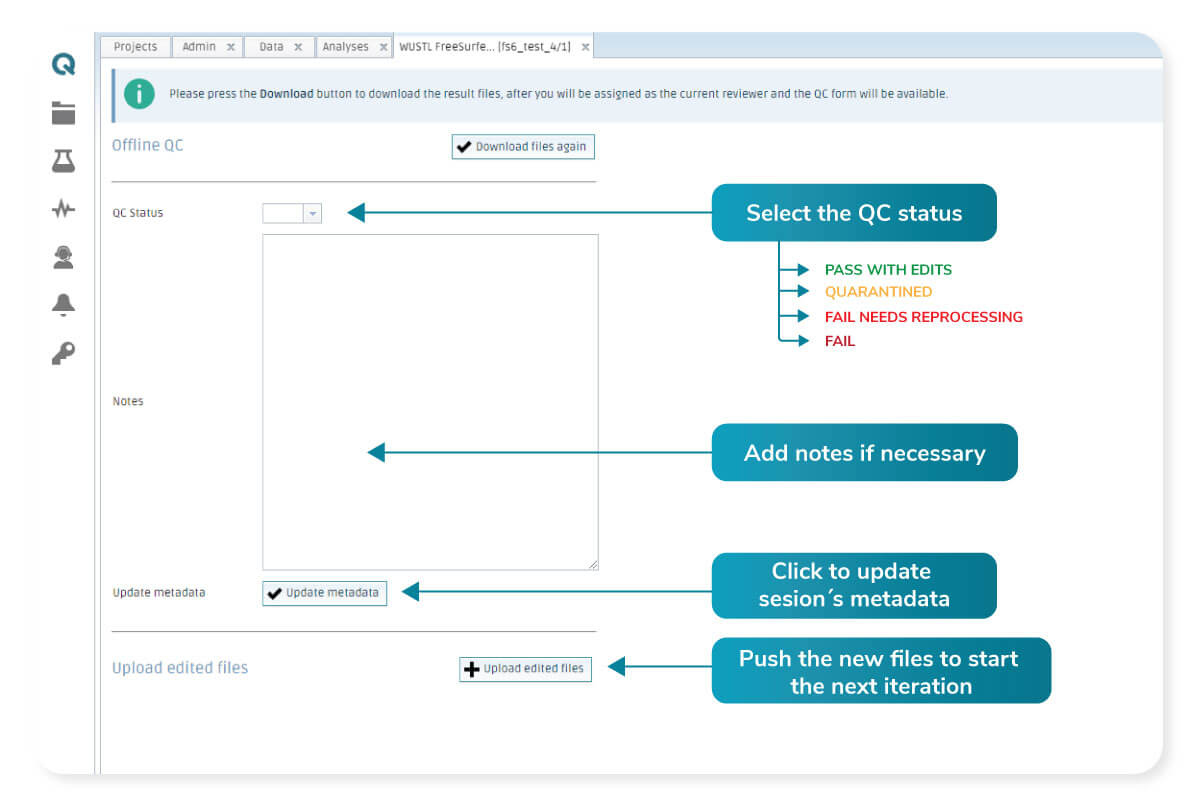
Platform interface allows you to update the different metadata values. Other’s are automatically set depending on the actions performed.
When the iteration has finished, the same results handling takes place. A button allows the user to download the new results and so repeat the quality control assessment on-premise. For this and the next iterations, the options shown will be slightly different:
5. PASS with edits: The results produced are meeting the expectations after being edited.
6. QUARANTINED: The processed data produced results that cannot be further improved by editing, and the session is going to be discarded.
7. FAIL needs reprocessing: The results can be tuned, and a new run is going to be performed using the edited files.
8. FAIL: The processed data produced results that cannot be further improved by editing, and the session is going to be discarded.
If option 7 is selected, a new iteration will start. If option 5 is selected, the pipeline will stop and the final results will be the ones produced.
Managing the iterative process
On top of this iterative process, the analysis updates some metadata values on the session being analyzed in order to control and assign the different parts of the process.
When the data is downloaded, a metadata field called “QC Assignee” is updated in the session. This will write the username of the person that has downloaded the results and is performing the quality control. This value allows the project administrator to control who is performing the quality check on each session of the project.
At the moment of selecting the quality control option (1 to 8), the session’s metadata field “Analysis Results Quality Control” is updated to the corresponding value. If any comment is added to the text input, a metadata field called “Quality Control Comments” is added with the information provided. When the user in charge of editing the results uploads the edited files, the metadata field “QC Assignee” is set to “None” as the next iteration begins.
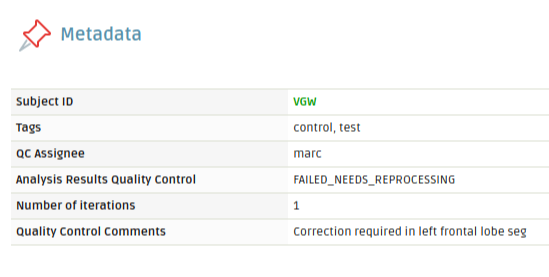
Subject session metadata updated after running the iterative tool in the platform.
Extra metadata fields could be added in order to control the process. The number of iterations can also be tracked using the session’s metadata, to know how many edits a particular session required.
Tools that can benefit from the process
Medical imaging tools that perform tissue segmentation, such as FreeSurfer, ANTs, lesion segmentation tools, can potentially increase their accuracy significantly when applying this process. Manual labeling is a time-consuming process and automated tools can save experts a lot of time; quality assessment and editing is much less of a burden, and the initial labels provided by such a tool would ease these tasks further. Other applications such as slice or plane selection, ROI placement, skull stripping could also benefit from using an iterative pipeline.
The value of the hybrid model can be taken even further than the research and analysis applications above. Executed in the QMENTA platform, this iterative process allows experts to combine the power of the cloud with any on-premise software specific to the individual use-case: think radiological viewers, oncology reading tools, and wider applications such as electrophysiological data annotation. Contact us to see how we can help you.
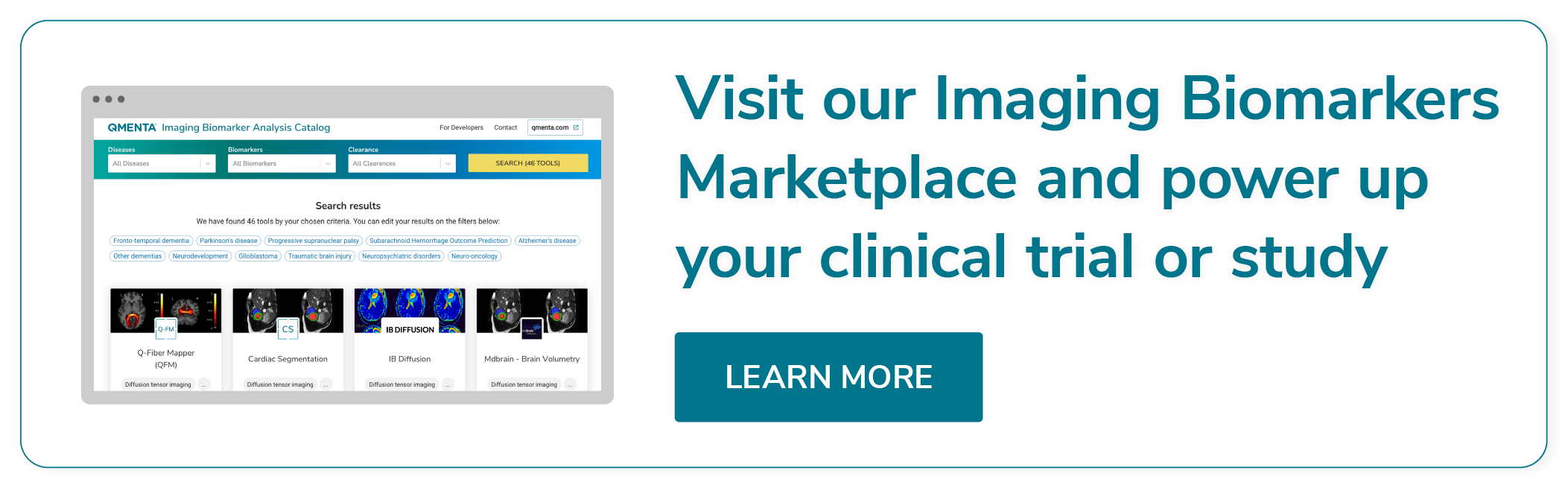