The World Health Organization estimates that around 450 million people suffer from psychiatric disorders. They predict that one in four people in the world will be affected by a problem related to their mental health at some point in their lives. This makes psychiatric disorders one of the leading causes of ill-health and disability worldwide. Even while being one of the most common pathologies in our society, the actual diagnosis is based on questions to the patient related to their symptoms, possible manifestations of the disease, and behavioral criteria. Therefore, doctors are only taking into account subjective information. As symptoms overlap between disorders, and sometimes patients are unable to properly answer the questions they are asked, an accurate diagnosis is very difficult to make.
What can you find in the brain?
Itis necessary to obtain an objective measure that can complement the subjective information obtained from the patient. A potential good source of biomarkers can be found in neuroimaging techniques, which are a non invasive way of obtaining information about the structure and functionality of the brain. The challenge is to figure out what information from these techniques can be informative for this concrete problem. For example, of the pictures below, could you say which one belongs to a patient suffering from a psychiatric disorder and which one belongs to a healthy control subject? Changes in structure and functionality are very subtle and doctors are not able to obtain a diagnosis just by looking at them. It is necessary to extract information that can help classify patients between suffering from a psychiatric disorder and healthy control individuals.
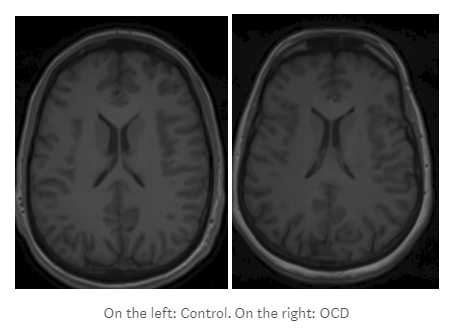
Why Deep Learning and not Machine Learning?
The approaches for psychiatric disorders classification on neuroimaging have changed compared to two decades ago. Before, analyses were focused on comparing patients with a specific disease against control individuals reporting neuroanatomical or neurofunctional differences at a group level. The problem with the proposed technique is that they assume different parts of the brain act independently, so the reported results are obtained by independent comparisons between voxels in the brain. However, studies show that several psychiatric and neurological symptoms are best explained by network-level changes in structure and function rather than focal alterations.
Machine Learning (ML) and Deep Learning (DL) techniques are multivariate methods, which means that a decision involves more than a single variable, and so, by their nature, they allow us to overcome problems with the previous analysis.
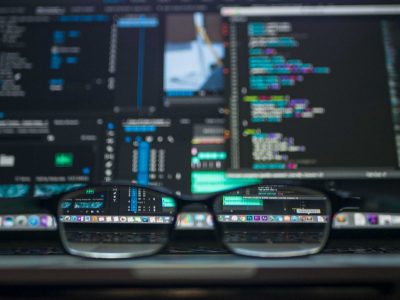
In the last decade, ML methods have been applied to classify different psychiatric illnesses. The most common classification algorithm applied is Support Vector Machine (SVM). One of the problems concerning MLtechniques, is the necessity of a feature selection process instead of applying the algorithm directly to raw data. Feature selection aims to eliminate high-correlated data that will decrease the performance of the model and input to the model relevant features. To perform a successful feature selection, it is necessary to have a good understanding of the data, which normally is a tedious work and requires a great amount of time.
In contrast, DL methods can be applied to raw data, without the necessity of a feature selection technique. Deep neural networks apply consecutively non-linear transformations to their inputs that allow the technique to extract useful information from raw data, generating features that are relevant to perform the final task without any human interaction in the process.
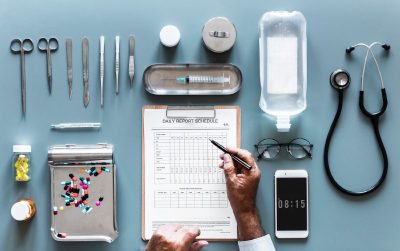
What does it mean for science?
Applying deep learning to the clinical diagnosis of mental health can have a great impact in the treatment of these disabilities. These techniques can be used to obtain biomarkers from brain images, which will lead to a better understanding of psychiatric disorders by measuring and evaluating specific characteristics of the brain, such as volume information from brain regions or connectivity information between areas. Those biomarkers can be used to improve the accuracy of the diagnosis, and consequently selection of a personalized treatment for each patient. Altogether, this can potentially reduce the impact of mental health problems in our society, or at least, improve the way doctors deal with them.
References.
http://www.who.int/whr/2001/media_centre/press_release/en/
Fox, M. D., Snyder, A. Z., Vincent, J. L., Corbetta, M., Van Essen, D. C., and Raichle, M. E. (2005). The human brain is intrinsically organized into dynamic, anticorrelated functional networks. Proceedings of the National Academy of Sciences of the United States of America, 102(27):9673–9678.
Haller, S., Missonnier, P., Herrmann, F., Rodriguez, C., Deiber, M.-P., Nguyen, D., Gold, G., Lovblad, K.-O., and Giannakopoulos, P. (2013). Individual classification of mild cognitive impairment subtypes by support vector machine analysis of white matter dti. American Journal of Neuroradiology, 34(2):283–291.
Rashid, B., Arbabshirani, M. R., Damaraju, E., Cetin, M. S., Miller, R., Pearlson, G. D., and Calhoun, V. D. (2016). Classification of schizophrenia and bipolar patients using static and dynamic resting-state fmri brain connectivity. Neuroimage, 134:645–657.
Vergara, V. M., Mayer, A. R., Damaraju, E., Kiehl, K. A., and Calhoun, V. (2017). Detection of mild traumatic brain injury by machine learning classification using resting state functional network connectivity and fractional anisotropy. Journal of neurotrauma, 34(5):1045–1053.